The purpose of machine learning programs is to analyze data, recognize patterns, and make predictions based on data. Machine learning algorithms enable the identification of patterns within data to facilitate accurate predictions and decision-making without explicit programming.
This technology is widely utilized in various sectors like image recognition, medical diagnosis, and financial analysis. By automating tasks, enhancing precision, and saving time, machine learning programs offer significant benefits. Other options, such as translating algorithms, determining algorithm constructions, and finding approximate solutions, differ in their objectives and functions.
In essence, the core purpose of machine learning is to extract insights from data, enabling continuous learning and improvement across different applications and industries.
Decoding Machine Learning Goals
Machine learning programs are designed to analyze large datasets, identify patterns, and make predictions based on the data. These programs utilize algorithms and statistical models to automate tasks, improve accuracy, and save time and resources in various applications such as image and speech recognition, medical diagnosis, and financial analysis.
The Core Objective: Predictive Analysis
The primary goal of machine learning programs is to analyze large data sets, recognize patterns, and make predictions based on the data. This process is known as predictive analysis and is achieved by using algorithms and statistical models. Machine learning programs can identify patterns that are not easily recognizable by humans, allowing for more accurate predictions and decision-making.
Predictive analysis is used in a variety of applications, including image and speech recognition, medical diagnosis, and financial analysis. By automating tasks, improving accuracy, and saving time and resources, machine learning programs are becoming increasingly important in today’s data-driven world.
Beyond Predictions: Other Key Outcomes
While predictive analysis is the core objective of machine learning programs, there are other key outcomes that they can achieve. These include:
- Classification: Machine learning programs can classify data into different categories based on patterns that are identified during the analysis process.
- Clustering: Machine learning programs can group similar data points together based on the patterns that are identified during the analysis process.
- Anomaly detection: Machine learning programs can identify data points that do not fit the expected pattern, which can be useful in fraud detection and cybersecurity.
- Recommendation systems: Machine learning programs can analyze user behavior and make personalized recommendations based on that analysis.
In conclusion, the purpose of machine learning programs is to analyze large data sets, recognize patterns, and make predictions based on the data. However, they can achieve other key outcomes such as classification, clustering, anomaly detection, and recommendation systems. By harnessing the power of machine learning, organizations can gain valuable insights from their data and make more informed decisions.
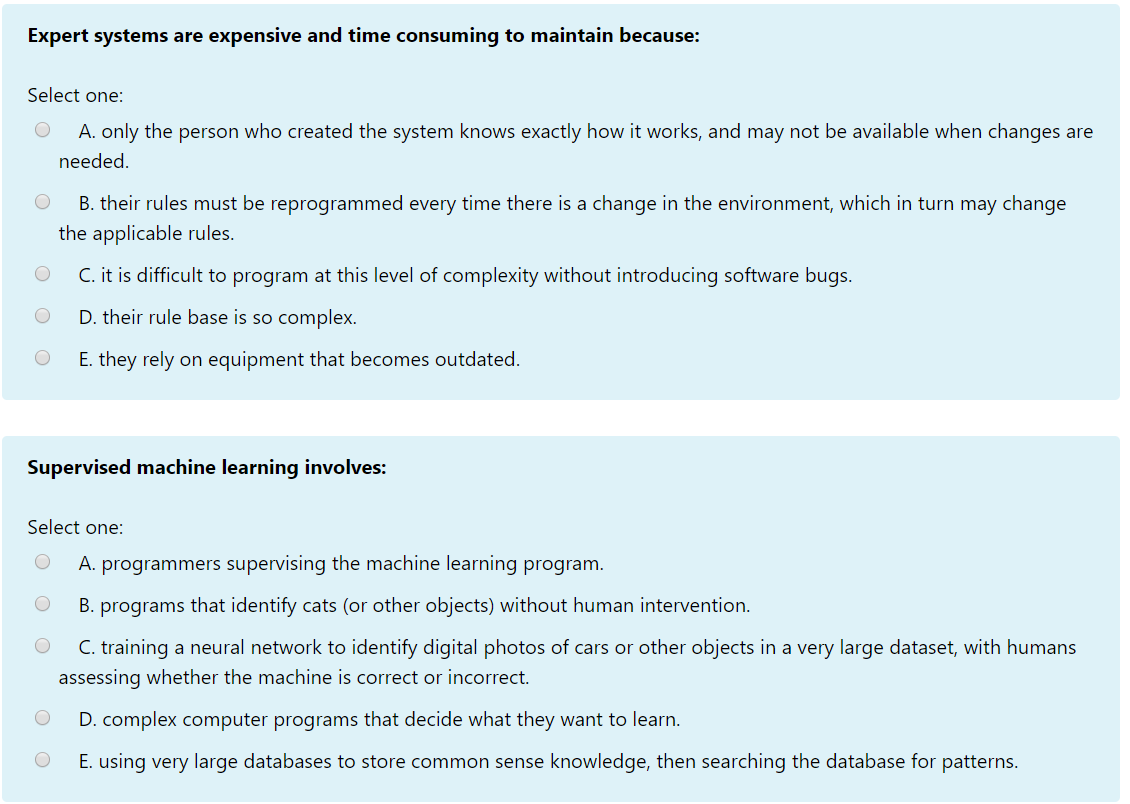
Credit: www.chegg.com
Pattern Recognition And Data Analysis
The purpose of machine learning programs is to analyze large data sets, recognize patterns, and make predictions based on data. These programs use algorithms and statistical models to identify patterns in data and automate tasks, improving accuracy and saving time and resources.
Other options, such as translating natural language or finding approximate solutions, describe different types of algorithms and purposes.
Unveiling Hidden Patterns
Machine learning programs excel at unveiling hidden patterns within large datasets. By employing complex algorithms and statistical models, these programs can identify intricate patterns that might not be apparent to human analysts. This capability is invaluable in various fields, including finance, healthcare, and marketing, where uncovering hidden patterns can lead to actionable insights and strategic decision-making.
Data-driven Decisions
One of the primary purposes of machine learning programs is to facilitate data-driven decisions. By recognizing patterns and trends within datasets, these programs empower organizations to make informed choices based on empirical evidence rather than gut instincts. This data-centric approach often leads to more accurate predictions and optimized strategies, ultimately enhancing operational efficiency and driving business success.
Machine Learning In Decision Making
Machine learning programs aim to analyze extensive data sets, identify patterns, and generate predictions based on the data. By utilizing algorithms and statistical models, they automate tasks, enhance accuracy, and save time across various fields like image recognition and financial analysis.
Automating Complex Decisions
Machine learning programs play a crucial role in automating complex decisions by utilizing algorithms and statistical models to analyze large datasets and identify patterns. By doing so, these programs can make accurate predictions based on the data without the need for explicit programming. This enhances efficiency and enables businesses to streamline decision-making processes.
Enhancing Human Judgment
Another key purpose of machine learning programs is to enhance human judgment. By leveraging advanced algorithms, these programs can assist humans in making informed decisions by providing valuable insights and analysis of complex data. This collaboration between machine learning and human judgment leads to improved decision-making and more effective outcomes.
Machine Learning Applications
Machine learning programs aim to analyze vast data sets, identify patterns, and generate predictions based on the data. By leveraging algorithms and statistical models, these programs automate tasks, enhance accuracy, and optimize resources in various fields such as image recognition, medical diagnosis, and financial analysis.
Transforming Industries
Machine learning applications are revolutionizing various industries by leveraging data to drive decision-making, automation, and innovation. Industries such as healthcare, finance, retail, and manufacturing are experiencing significant transformations through the implementation of machine learning programs.
Case Studies: Real-world Impact
Real-world case studies vividly demonstrate the impactful nature of machine learning applications. For instance, in healthcare, machine learning algorithms have been employed to analyze medical images, aiding in early disease detection and improving patient outcomes. Similarly, in the financial sector, predictive analytics powered by machine learning have enhanced fraud detection and risk management, safeguarding financial institutions and their customers.
Comparing Machine Learning Objectives
Machine learning programs are designed to analyze vast datasets, identify patterns, and generate predictions based on the data. By leveraging algorithms and statistical models, these programs automate tasks, enhance accuracy, and save time across various applications like image recognition and financial analysis.
A Versus B
Machine learning programs are designed primarily to analyze large data sets, recognize patterns, and make predictions based on data. This involves using algorithms and statistical models to identify patterns in data and make decisions without explicit programming.
C Versus D
The purpose of machine learning programs is not to automatically translate algorithms from natural language to machine language (Option B) or to determine whether an algorithm can be constructed to answer “yes” or “no” for all possible inputs (Option C). Instead, it is to find approximate solutions to problems that would otherwise require an unreasonably long amount of time to solve (Option D).
Future Directions In Machine Learning
Machine learning programs aim to analyze data, identify patterns, and predict outcomes based on the information available. By using algorithms and statistical models, these programs automate decision-making processes without explicit programming, leading to improved efficiency and accuracy in various fields like image recognition and financial analysis.
Innovations On The Horizon
Machine learning is rapidly evolving, and several innovations are expected to shape its future. One such innovation is the advancement of deep learning techniques to enable more complex and accurate predictions. Additionally, the integration of AI with edge computing is anticipated to enhance real-time decision-making and reduce latency in processing. The development of unsupervised learning algorithms is another area of focus, aiming to enable machines to learn from data without labeled responses. These innovations are poised to revolutionize industries such as healthcare, finance, and autonomous vehicles, opening up new possibilities for machine learning applications.
Ethical Considerations And Challenges
As machine learning continues to advance, ethical considerations and challenges come to the forefront. The ethical implications of AI and machine learning algorithms raise concerns about privacy, fairness, and accountability. With the potential for bias in algorithms and the misuse of personal data, addressing these ethical challenges is crucial. Moreover, the transparency and interpretability of machine learning models are essential to ensure that decisions made by these systems are understandable and justifiable. As the technology continues to advance, it is imperative to establish ethical frameworks and regulatory standards to govern the responsible development and deployment of machine learning programs.
Frequently Asked Questions
What Best Describes The Purpose Of Machine Learning Programs?
Machine learning programs analyze data, identify patterns, and make predictions based on the information processed.
What Is The Main Goal Of Machine Learning?
The main goal of machine learning is to analyze data, identify patterns, and make predictions based on the data.
Which Of The Following Statements Best Describes Machine Learning?
The purpose of machine learning programs is to analyze large datasets, recognize patterns, and make predictions based on the data. Machine learning uses algorithms and statistical models to identify patterns in data and make decisions without explicit programming. This technology is used in various applications, including image and speech recognition, medical diagnosis, and financial analysis.
Machine learning’s core ability is to iteratively learn and improve from data over time, making it essential in diverse fields and everyday applications.
What Is The Machine Learning Used For?
Machine learning is used to analyze large data sets, recognize patterns, and make predictions based on data. This allows for automated tasks, improved accuracy, and resource savings in various applications such as image and speech recognition, medical diagnosis, and financial analysis.
What Is The Main Purpose Of Machine Learning Programs?
Machine learning programs analyze data, detect patterns, and predict outcomes to automate decision-making processes.
Conclusion
Machine learning programs excel in analyzing data, recognizing patterns, and making accurate predictions based on the information available. By leveraging algorithms and statistical models, these programs automate tasks, enhance precision, and optimize resource management. This transformative technology is widely used in various sectors for improved efficiency and decision-making capabilities.
Leave a Reply